AI utilizes advanced algorithms and predictive analytics to process vast amounts of customer data, leading to valuable insights and enhanced personalization. By leveraging AI, companies can now uncover hidden patterns and trends that were previously inaccessible in large datasets.
By leveraging AI to analyze customer data, companies can significantly enhance customer experiences and relationships. AI excels at detecting patterns and deriving meaning from large, complex datasets. This enables businesses to derive actionable insights from customer behaviors, preferences, and motivations.
Table of Contents
Customer data analysis is being revolutionized by Artificial Intelligence (AI).
AI empowers organizations to personalize interactions, predict customer data analysis needs, understand sentiment, improve products and services, and optimize marketing campaigns. Some of the key capabilities of AI for customer insights include:
- Personalization – Create customized recommendations and experiences for each customer
- Predictive analytics – Forecast future behaviors and trends to proactively meet customers’ needs
- Sentiment analysis – Detect how customers feel about products, services, and brands
- Chatbots – Enable natural conversations to assist and engage customers
- Fraud detection – Identify abnormal behaviors and suspicious activities
- Customer segmentation – Divide customers into groups based on common attributes to tailor engagements
With the rise of big data and increased computing power, AI will continue growing as an indispensable tool for businesses seeking to better understand their customers. By harnessing AI-generated customer insights, companies can foster stronger customer relationships, boost satisfaction, and gain competitive advantages.
Data Collection
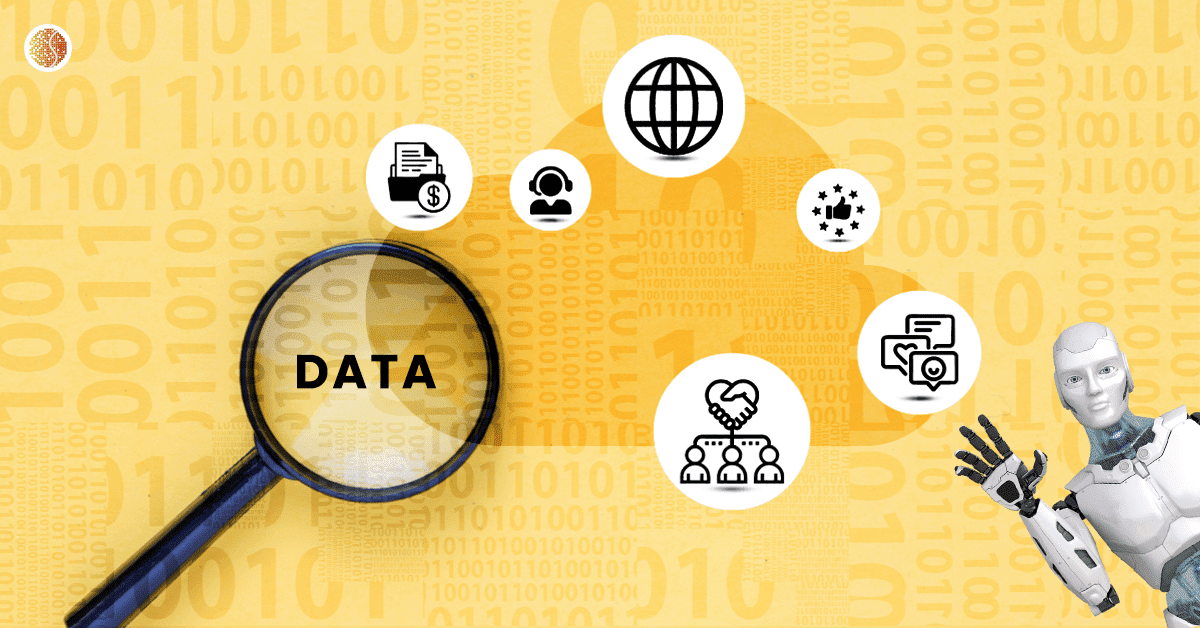
Companies today have access to more customer data analysis than ever before. This data comes from a variety of sources and can provide valuable insights into customers when processed and analyzed properly. Here are some of the main types of customer data collected and methods for gathering it:
Transactional data: This includes any data related to customer purchases, both online and in stores. Retailers can analyze details like items purchased, prices, purchase frequency, returns, etc.
Web data: Visitor activity on a company’s website provides a wealth of information. Web analytics tools can track everything from pages visited to buttons clicked. Marketers can see how visitors navigate sites and where they encounter friction.
Social media data: Posts, shares, comments, and other social interactions give insight into customers’ interests, influencers, and sentiment towards brands. Social listening tools gather this data across platforms.
Customer service interactions: Records of email, phone, live chat, and other customer inquiries show common questions, complaints, praise, suggestions, and more. Analyzing this data helps improve future support.
Reviews: Customer reviews on a company’s own website as well as third-party sites like Yelp reveal satisfaction levels, product/service feedback, and reputation. Brands can track and respond to reviews over time.
Loyalty data: For companies with loyalty programs, member profiles and activity provide a rich customer dataset. This can include demographic info, purchase history, engagement levels, special offers redeemed, and real-time location data.
CRM data: Customer relationship management systems centralize various data streams to create unified customer profiles. This facilitates more personalized and impactful marketing.
Of course, privacy is always a top concern when gathering customer data. Companies must be transparent in disclosing what is collected and how it will be used. Consent should be obtained where applicable. Customer Data analysis must be handled securely and only utilized for legitimate business purposes. With the proper measures in place, customer data can deliver powerful AI-driven insights.
Data Processing
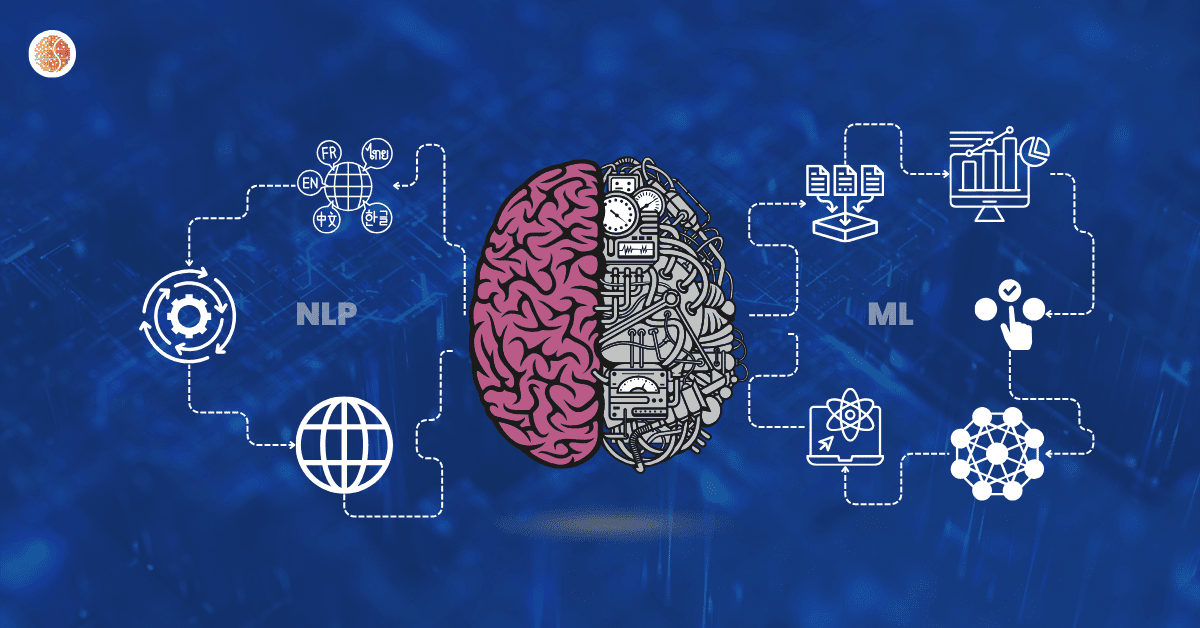
AI and machine learning are transforming how businesses process and analyze customer data. Whereas traditional data analytics relied on rules-based programming, AI systems can process natural language, image, voice, and sensor data using neural networks modeled after the human brain. This allows for more nuanced analysis.
Two key AI techniques for processing customer data are:
Natural Language Processing (NLP): NLP algorithms can extract insights from open-ended customer surveys, support tickets, product reviews, social media, and call center logs. By understanding sentiment and meaning, businesses can better grasp customers’ wants and frustrations.
Machine Learning: Machine learning algorithms uncover patterns in customer data that humans would likely miss. As the algorithms are exposed to more data, they become better at predicting customer needs and behaviors. Machine learning excels at powering recommendation engines, predicting churn, personalizing messaging, and automatically tagging content.
Together, NLP and machine learning enable businesses to derive unprecedented insights from massive amounts of unstructured customer data. Rather than relying solely on what customers say they want, companies can understand what customers actually want based on their behaviors, conversations, and contexts. This data-driven approach leads to more delightful customer experiences through hyper-personalization.
Using AI to Personalize the Customer data analysis Experience
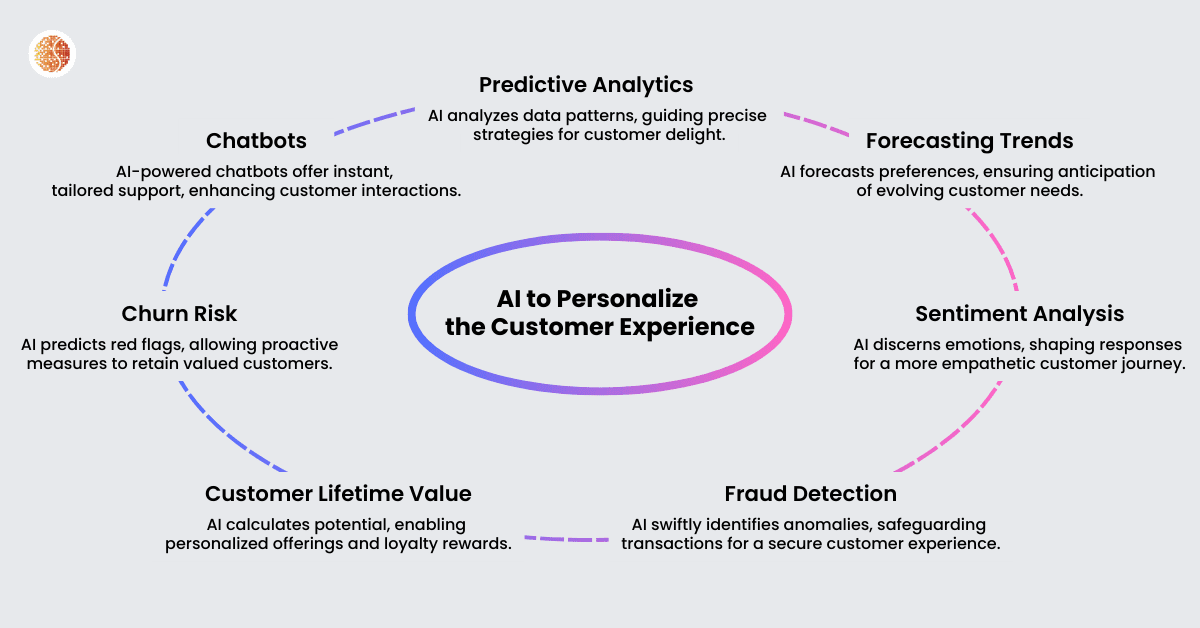
Brands today have access to more customer data than ever before. Every online interaction generates valuable insights into customer preferences, behaviors, and motivations. Artificial intelligence allows companies to harness this data to create hyper-personalized experiences.
Recommendation engines powered by AI analyze customer data analysis histories and patterns to provide tailored suggestions. For example, Netflix and Amazon use algorithms to recommend specific movies and products based on each user’s unique tastes. Rather than relying on generic promotions, brands can pinpoint exactly which items each customer is most likely to enjoy.
Beyond product recommendations, AI enables customization across the customer data analysis journey. Chatbots can be designed with natural language processing to hold personalized conversations at scale. Dynamic websites can change content or offers for each visitor in real time based on their profile and past behavior. Email and social ad targeting lets marketers reach the right customers with relevant messages.
Powerful segmentation enabled by AI allows brands to precisely identify the needs of each micro-market. Customers expect experiences tailored just for them. With artificial intelligence, companies can gain granular insights to deliver personalized interactions that drive satisfaction, engagement and loyalty.
Predictive analytics are techniques that leverage historical data and machine learning algorithms to identify patterns and make predictions about the future. This allows businesses to have deeper insight into customer behavior and preferences. With predictive analytics, businesses can forecast trends, understand customer lifetime value, and assess churn risk.
Businesses can use predictive customer data analysis to uncover trends in customer purchases, website visits, product interests, and more. By analyzing historical data, predictive models can spot patterns and project those forward. For example, retailers may predict future sales numbers for a given product line based on past sales data and seasonality trends. Media companies may forecast website traffic growth over the next year. Predictive analytics provides data-driven insight into where things are headed.
Calculating customer lifetime value (LTV) involves estimating the total revenue a business can expect from a customer over the duration of their relationship. This helps prioritize marketing efforts on high-value customers. Predictive analytics assesses past purchase history and projects expected buying patterns into the future. This accounts for frequency of purchases, churn risk, projected growth in spending, and other factors. High LTV customers can be targeted for retention while low LTV ones may be deprioritized.
Businesses hate losing customers, and predictive analytics can identify those most at risk of churning. By assessing past churn rates and comparing behavioral patterns against current customers, models can predict who is likely to cancel or stop buying. Businesses can then develop targeted campaigns to re-engage customers with high churn scores, saving those relationships. This allows retention programs to focus on customers companies can least afford to lose. Predictive analytics powers proactive churn reduction.
The ability to understand and analyze customer attitudes and emotions from their feedback and interactions is extremely valuable for brands. Sentiment analysis is the use of natural language processing, text analysis, and computational linguistics to systematically identify, extract, and quantify affective states like attitudes, emotions, and subjective evaluations.
Brands can apply sentiment analysis to product reviews, social media conversations, customer support tickets, emails, chat logs, and survey responses to gain actionable insights. For example, a hotel chain may analyze customer tweets to their handle and conversations in travel forums to gauge public perception of their brand and specific properties. Or an ecommerce retailer can use sentiment analysis on product reviews to identify their most beloved and maligned products.
Advanced sentiment analysis AI can go beyond simple categorization of positive, negative, and neutral sentiment. The AI can detect more nuanced emotions like joy, sadness, anger, disgust, and more. It can also analyze text for subjectivity, intentions, and personality traits. When applied across massive datasets, brands can uncover invaluable insights like which customer segments have the highest brand loyalty and satisfaction. They can also monitor how campaigns, new products, and events impact different demographics’ brand sentiment over time. The AI continues learning and improving analysis accuracy the more customer data it processes.
With these kinds of customer insights, brands can track campaign success, identify brand advocates and detractors, gauge market reception to new products, improve customer service, and build deeper customer relationships. Sentiment analysis is a powerful AI innovation that allows brands to listen to the voice of the customer at scale.
Chatbots
AI has revolutionized customer engagement through chatbots. Brands of all sizes are adopting conversational AI to automate customer service and sales interactions.
AI-powered chatbots can understand natural language, hold fluid conversations, access customer data, and resolve common queries instantly. This enables them to handle enormous volumes of customer inquiries without needing to staff large call centers.
Chatbots excel at tasks like order tracking, account lookups, FAQs, and appointment scheduling. They free up human agents to handle more complex issues requiring emotional intelligence and discretion.
- Beyond customer service, chatbots are being used across the customer journey:
- Lead generation – Chatbots can qualify leads 24/7 by asking questions and routing prospects to sales reps.
- Conversions – Many brands use chatbots to nurture leads towards a purchase through personalized messaging at scale.
- Post-purchase – Chatbots can automate order confirmations, shipping updates, returns, and other routine purchase interactions.
- Upselling & cross-selling – Using predictive analytics, chatbots can recommend complementary products and tailor offers to each customer.
AI chatbots are an invaluable addition to any customer engagement strategy. With their ability to deliver instant, personalized and scalable conversations, they satisfy customers’ growing expectations for always-on service and interactions.
Fraud Detection
With the rise of e-commerce, online fraud has also increased. AI can help detect fraudulent transactions and suspicious patterns in customer behavior.
Fraud prevention systems analyze large volumes of transaction data in real-time to identify signals of potential fraud. They build profiles of legitimate customer behavior through machine learning, and detect anomalies that deviate from the norm.
Some common signs of fraud AI might detect:
- Drastic changes in spending patterns
- Transactions from new devices or unfamiliar locations
- Multiple transactions in quick succession
- Suspicious information submitted during account creation
When a potentially fraudulent transaction is flagged, the system can automatically block the transaction or trigger additional identity verification. This protects both the business and legitimate customers from fraud losses.
AI fraud detection is powered by advanced techniques like neural networks, clustering, and decision trees. These can uncover complex relationships within data that humans may miss. The algorithms also get smarter over time as more customer data is accumulated.
Proactive fraud prevention using AI saves companies significant money compared to manual reviews. It also provides customers with a more seamless experience, avoiding unnecessary friction from false positives. With the help of AI, businesses can stay one step ahead of the latest fraud tactics and patterns.
Customer Segmentation
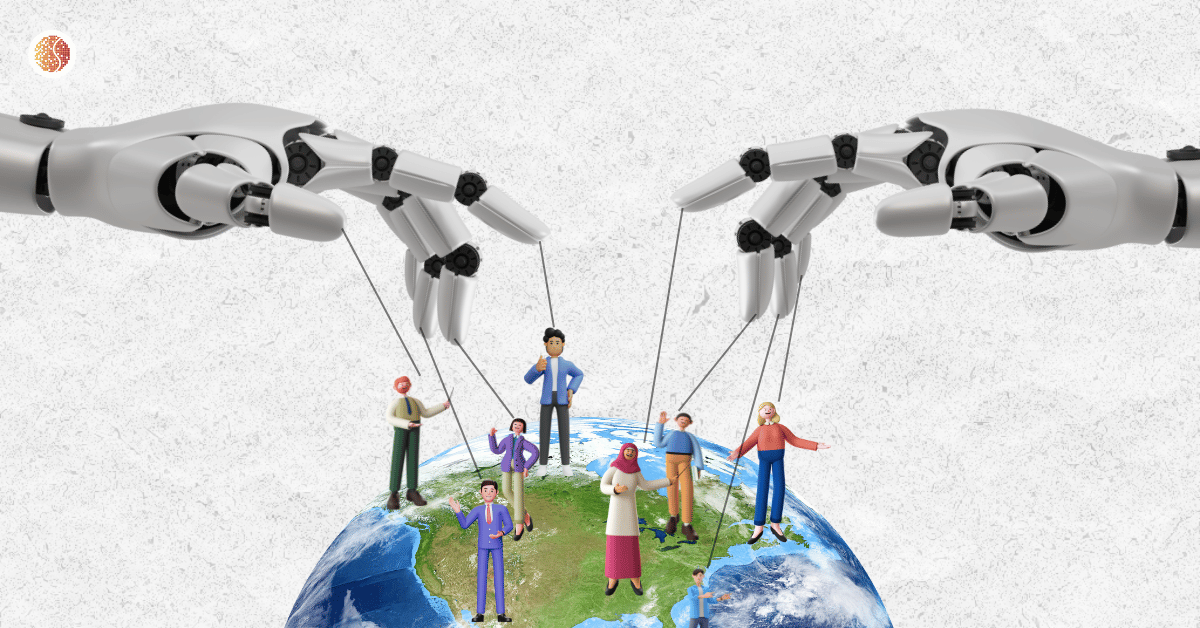
Customer segmentation allows businesses to group customers based on common attributes and behaviors. This enables companies to tailor products, messaging, offers and experiences to best meet the needs and interests of each customer segment.
Some key ways businesses leverage customer segmentation include:
Demographic segmentation – grouping customers by attributes like age, gender, income, education level, occupation, marital status, household size etc. This helps create targeted marketing campaigns.
Geographic segmentation – dividing customers by location, climate, population density etc. Useful for localization strategies.
Behavioral segmentation – categorizing customers based on behaviors like purchase history, channel usage, spending habits, usage frequency, loyalty status etc. Enables personalized recommendations.
Psychographic segmentation – segmenting by personality traits, attitudes, interests, lifestyles and values. Valuable for fine-tuning brand messaging.
Needs-based segmentation – grouping by common needs, problems, desires or product features sought. Allows for needs-focused product development.
Value-based segmentation – categorizing by customer value metrics like profitability, lifetime value, purchase frequency etc. Helps prioritize high-value customers.
Hybrid segmentation – using a combination of different segmentation approaches for deeper insights. Provides a well-rounded view of customers.
Advanced analytics tools empower businesses to gain data-driven customer insights from segmentation. Machine learning can even enable autonomous customer micro-segmentation for hyper-personalization. Overall, customer segmentation is a powerful technique for understanding customers and serving them better.
Conclusion
Artificial intelligence has the potential to transform how companies understand their customer data analysis and offer valuable insights. By leveraging vast amounts of data, AI can reveal hidden patterns, identify customer segments, and deliver personalized recommendations and experiences.
AI-powered solutions are continuing to evolve alongside increases in data volume and machine learning capabilities. As more companies adopt AI for customer insights, they should keep a few best practices in mind:
- Focus AI efforts on clear business objectives and impactful use cases to drive ROI
- Maintain high data quality and integrity to train accurate machine learning models
- Prioritize transparency, ethics and privacy when collecting and analyzing customer data
- Combine AI with human oversight and expertise for optimal results
- Start small, test quickly, and iterate – AI projects require an agile, experimental approach
Looking ahead, AI will provide ever deeper insights into the customer data analysis journey across touchpoints. With proper strategy and governance, companies can unlock immense value in customer data and build stronger engagement, loyalty and relationships. AI represents an exciting new frontier in understanding customers and serving them better.